We wanted to take this concept further, and visualize what the human eye sees from EEG data. You can read the paper we published here, but that’s not the point. I noticed that all the projects we were working on were time crunched and were never replications of other neuroscience papers.
Our lab operated on “90 minute sprints”, a method inspired by MIT’s lab (Our boss was a professor at MIT before this).
If any results came out of a project before 90 min, we would keep working on it in the next sprint. If there were no results, we would abandon the project and move to something else.
This high intensity work flow was great for developing deep CS + hardware understanding, but there was always a voice in the back of my head that would question how valuable they were.
Microsoft wasn’t built in 90 minutes, Tesla wasn’t built in 90 minutes, PayPal wasn’t built in 90 minutes, CRISPR wasn’t discovered in 90 minutes. We were trying to 1) Make breakthroughs 2) Make them quickly, which seemed impossible.
So I started looking into the incentive structure for research facilities and the outcome on the quality of research generated.
Academic incentives are messed up.
What I found was that research facilities across North-America have an incentive structure that doesn't promote good quality research.
Scientific Status
- Novelty and constant ground-breaking progress brings higher scientific status than long term research or exhaustive studies.
- Short term projects bearing little fruit aren't as risky as long term projects bearing little fruit.
Replication
- Replication + validation of previous work is discouraged.
- Independent, direct replications of others’ findings can be time-consuming for the replicating researcher.
- Replications are likely to take energy and resources away from other projects that reflect one’s own original thinking.
- Replications are generally harder to publish (because they’re viewed as being unoriginal).
- Even if replications are published, they are likely seen as ‘bricklaying’ exercises, rather than as major contributions to the field.
Validation
- Hypothesis validation brings more social clout than hypothesis invalidation. Ex: imagine spending 30 years on a long term study of cancer to realise your hypothesis for a cure isn’t viable. This is a big fear for researchers.
- Research that isn’t successful at supporting a hypothesis gets kept away in the file cabinet, which causes a lack of transparency in the space.
Criticism
- Criticism of the current academic incentive system only hurts your reputation.
- Researchers who stand up for true science and complain about “fake” research are perceived as being incompetent enough to succeed in the current hierarchy. They are seen as complainers who aren’t well suited enough for proper scientific study.
- Ex: “Princeton University psychologist Susan Fiske drew controversy for calling out critics of psychology. She called these unnamed “adversaries” names such as “methodological terrorist” and “self-appointed data police”, and said that criticism of psychology should only be expressed in private or through contacting the journals. Columbia University statistician and political scientist Andrew Gelman, “well-respected among the researchers driving the replication debate”, responded to Fiske, saying that she had found herself willing to tolerate the “dead paradigm” of faulty statistics and had refused to retract publications even when errors were pointed out. He added that her tenure as editor has been abysmal and that a number of published papers edited by her were found to be based on extremely weak statistics; one of Fiske’s own published papers had a major statistical error and “impossible” conclusions.”
As a result, the current research space has become saturated with short term projects with inflated results.
The number of faculty positions in the research space has remained in proportion with population growth, but cumulative PhDs awarded has not remained in proportion to faculty positions.
More PhDs are being handed out then ever before. This could either mean that 1) Researchers are getting much better at picking interesting topics to pursue and are finding much more concrete data supporting their thesis’s (could be due to technological advancement). 2) Researchers are inflating results to get their hands on PhDs.
The first could be true in an economy that’s rapidly growing with tech. The more competent the tech, the more data could be gathered supporting a hypothesis. Plus, the more economically incentivized research would become due to it’s high demand, which could explain the significant rise in PhDs.
GDP is the best measure of economic growth.
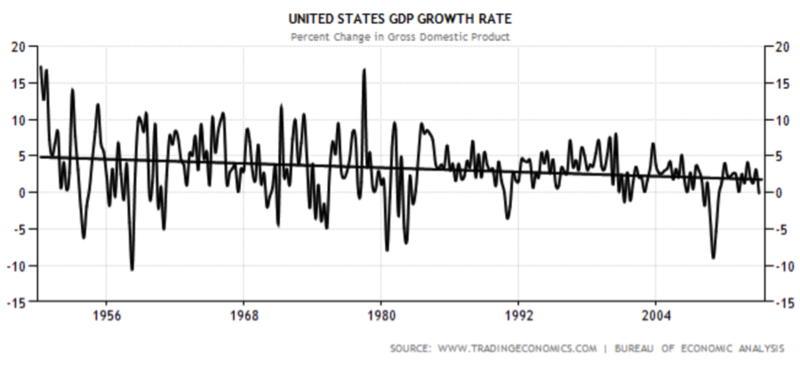
But growth is shrinking over time, which disproves the first hypothesis and further proves the second.
Troubling Stats
More than 50% of researchers have use >1 questionable work practice (QRPs)
Examples of QRPs
- selective reporting or partial publication of data (reporting only some of the study conditions or collected dependent measures in a publication)
- optional stopping (choosing when to stop data collection, often based on statistical significance of tests)
- p-value rounding (rounding p-values down to 0.05 to suggest statistical significance)
- file drawer effect (nonpublication of data)
- post-hoc storytelling (framing exploratory analyses as confirmatory analyses)
- manipulation of outliers (either removing outliers or leaving outliers in a dataset to cause a statistical test to be significant).
This has had major cumulative effects on the ability to replicate other papers:
“According to a 2016 poll of 1,500 scientists reported that 70% of them had failed to reproduce at least one other scientist’s experiment (50% had failed to reproduce one of their own experiments).”
It’s especially present in fields that fall subject to pseudo-science, like psychology and neuroscience (I remember altering some of the sample data I was gathering to make it fit my hypothesis back at the lab. I only did it for one test, but it was definitely tempting later on).
Psychology
Replicating trials in 2012 were done to see if older psychology papers could be replicated.
- 91% of new papers with author overlap from previous papers could be replicated
- 64% of those without couldn’t
Another set of replicating trials in 2015 examined the reprehensibility of the top 100 psychology papers from top science journals.
- 36% had significant findings compared to the 97% stated in the journal
When you look deeper into psychology, different sub-fields have different replication results.
- Cognitive psychology replication rate = 50%
- Social psychology replication rate = 25%
Papers with multiple researchers on board yield better replication results
- 91.7% with multiple researchers on board
- 64.6% without
Basically, older authors have more legitimacy and have a reputation to preserve, newer authors don’t. Plus, fields that rely on low amounts concrete data to make assumptions are probably not replicatable.
When there are multiple researchers on board, they keep each other accountable and their work yields more accurate results.
Why is it happening now?
Like mentioned before, the incentive structure for researchers doesn’t encourage paper replication and exhaustive or long term studies. The current “Publish or Perish” mentality is synchronised with the “Move fast and break things” mentality of startups.
The field is saturated with ideas and hypothesis’, but no one’s willing to put in the long hours to validate or invalidate them, so shortcuts are taken (inflated results and false positives).
This wasn't a problem 50 years ago. It only became a problem because:
- Big companies have been progressively substituting expensive and timely in-house research for university contracted research, to even cheaper and faster 3rd party research companies. Problem: companies want results reinforcing the use of their product. 1) creates bias towards validating hypothesis and not disproving them. 2) research companies in competition fight to bring companies the best and fastest results, cutting corners in the process.
- As the scientific community becomes more dense, it becomes harder to stand out. Without groundbreaking results, it’s very difficult to seem legitimate and competent in your field.
- There is an increasing stress on fast paced development. There are much fewer long term studies being executed on (relative to scientific community size) than there were in the 1950s. Global access and competition accelerates development but also squashes patience and validity.
What we can do about it
There doesn’t seem to be one solution. But, if you break down how a research paper is made, you can identify key areas where researchers throw in their biases.
Here are a few ways to tackle the problem:
- Tackling publication bias with pre-registration of studies→pre-determine and peer review your method for data collection / validation. Incentivises follow through and publication in the case of results contradicting hypothesis, and outcome alteration (QRPs)
- Emphasise replication attempts in teaching
- Reduce the p-value required for claiming significance of new results
- Address the misinterpretation of p-values
- Encourage larger sample sizes
- Share raw data in online repositories
- Fund replication studies specifically
- Emphasise triangulation, not just replication→attempt other methods challenging the problem and weigh different outcomes for each
I see pre-registration of studies and sharing data online in large repositories as some of the best ways to face the problem right now. I'm not sure how we'll get around it, but faulty research is laying down a foundation of sand and will cause huge long term problems if we don't find a way to restructure incentives.
If you want to learn more, check out this podcast with Peter Thiel and Eric Weinstein.